Computer Vision and the Path to an Enhanced AI Strategy
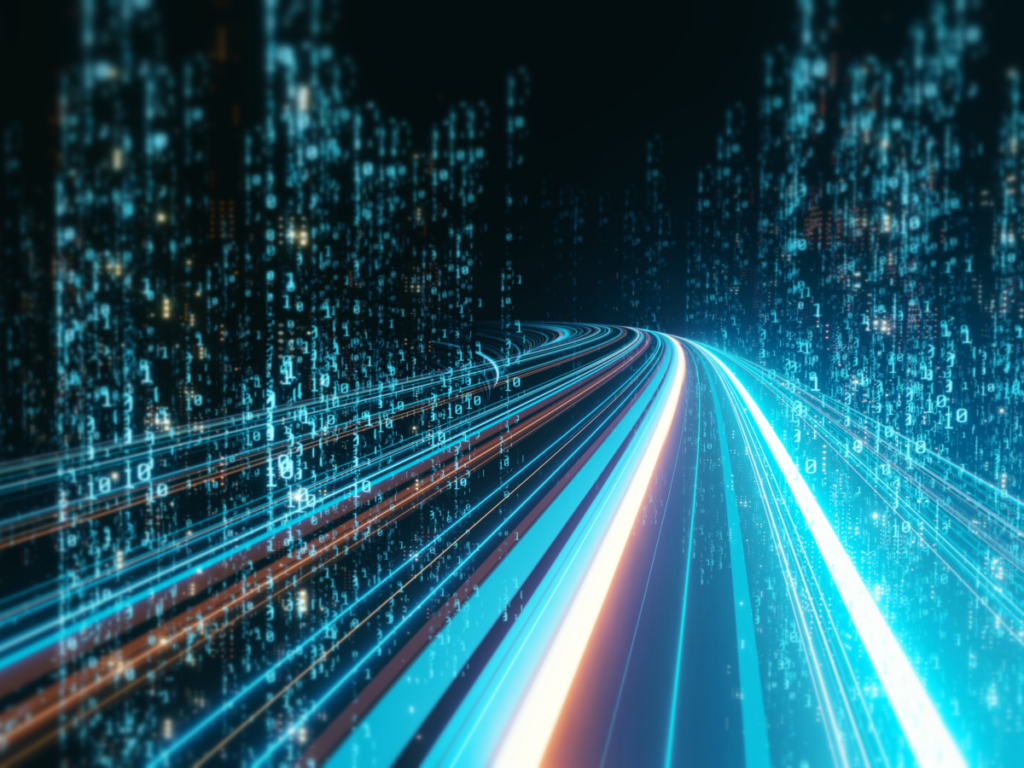
Computer vision provides unique opportunities for utilities to enhance grid insights, streamline operations, and improve customer experiences. Access to advanced model-building tools, cloud-managed services, and updated frameworks has revolutionized image analytics approaches. Yet, establishing a successful computer vision program poses challenges, particularly in sustaining value post-investment. A holistic approach is crucial, considering foundational, organizational, and financial aspects, to ensure a robust AI strategy.
Choose the Approach That Fits Your Needs and Budget
When considering investing in computer vision, groups often debate between “build” and “buy.” While managed services from major cloud platforms like AWS and Azure make building models quicker, they may lack domain-specific foundational models, tuning ability, and out-of-the-box functionality for data augmentation. Buying can be pricier upfront and lead to integration issues, but having modeling experience can greatly improve model performance, especially in tricky image analytics. It’s crucial to choose the approach that offers the most value for the specific problem.
When planning projects involving image analytics technology, it’s important to consider ML Operations and integration challenges. As projects scale, it becomes vital to have systems in place to monitor model versions, ensure data is usable, and operate at scale. By addressing these factors in project planning, you can ensure that the investment in image analytics delivers tangible value.
Measure ROI Early
Building baseline models and measuring relevant metrics early lays a foundation for representing the value of the investment. Machine learning is an experimental and iterative process, and we need to understand the incremental value gained with improved performance. When evaluating standardized model metrics like accuracy, precision, and recall, it’s important to ask the question “How good is ‘good enough?’” Strategically, it helps to think about what the model is accomplishing.
Image analytics is generally replacing human-in-the-loop processes, so a natural next question would be “What is human performance versus model performance on this specific task?” A small investment in a blind human QC effort in LabelOps processes can inform quality of a labeled dataset and help answer the question of whether to invest in data quality or model performance—and answer the question “How good is ‘good enough’ for this problem?”
Enable Velocity, Enable Reuse, Enable Value
Establishing a solid foundation of governance and enablement is critical for ensuring that computer vision deployment aligns with the organization’s strategic objectives and with ethical considerations.
Data Products and Consumption
As teams develop models, it’s strategically important to make data and model products available to the broader organization. For example, an image detection and classification model for utility assets would have downstream value for multiple teams and use cases. To avoid other teams re-inventing the wheel, large organizations should invest in broader data foundations where seamless discovery and adoption can take place.
Enablement
To integrate computer vision successfully, utilities must invest in the necessary infrastructure, provide training for staff to develop the requisite skills, and foster a culture that embraces innovation and continuous improvement. Enablement ensures that the organization not only adopts computer vision technology but is also prepared to leverage it to its full potential. This can help bridge the gap between data scientists and ML engineers and help teams get new models to production faster.
Cross-Functional Teams
Computer vision teams should include representation from data science, IT, operations, and other relevant departments, working under a shared vision and clearly defined objectives that align with the company’s broader goals. Encouraging open communication and regular meetings can maintain team alignment and ensure that everyone is moving in the same direction.
Stakeholder Engagement and Change Management
Decision makers are often hesitant to trust outputs from AI models. Change management is a key part of adoption and gaining value from the overall solution. One method that has proven effective is to develop interactive tools to help users understand model outputs next to the data. Integrating model outputs into decision-making tools early lets stakeholders get used to visualizing the data and providing feedback on model outputs to share ownership of the models alongside the technical team.
Unlocking the Future
Utility companies are uniquely positioned to leverage the power of computer vision in meeting—and exceeding—modern expectations of efficiency and innovation. By embracing a holistic AI strategy that encompasses foundational governance, cross-functional synergies, and a focus on maximizing data science investments, utilities can unlock considerable value. In doing so, they pave the way for enhanced operational processes, elevated customer experiences, and robust advantages in a future where AI-driven solutions stand at the forefront of industry innovation.
About the Author
Alex Johnson is a Lead Developer in Logic20/20’s Advanced Analytics practice.
Logic20/20 is a Solution Provider Member of UAI and a sponsor of Utility Analytics Summit 2024.
See Alex in person at Utility Analytics Summit 2024 April 9th-11th in Nashville, TN: Enhancing Your AI Strategy with Computer Vision.
Utility Analytics Summit 2024 is brought to you by Utility Analytics Institute and is produced by Endeavor Business Media