Data Hubs Surpass Data Lake Functionality for Asset IoT
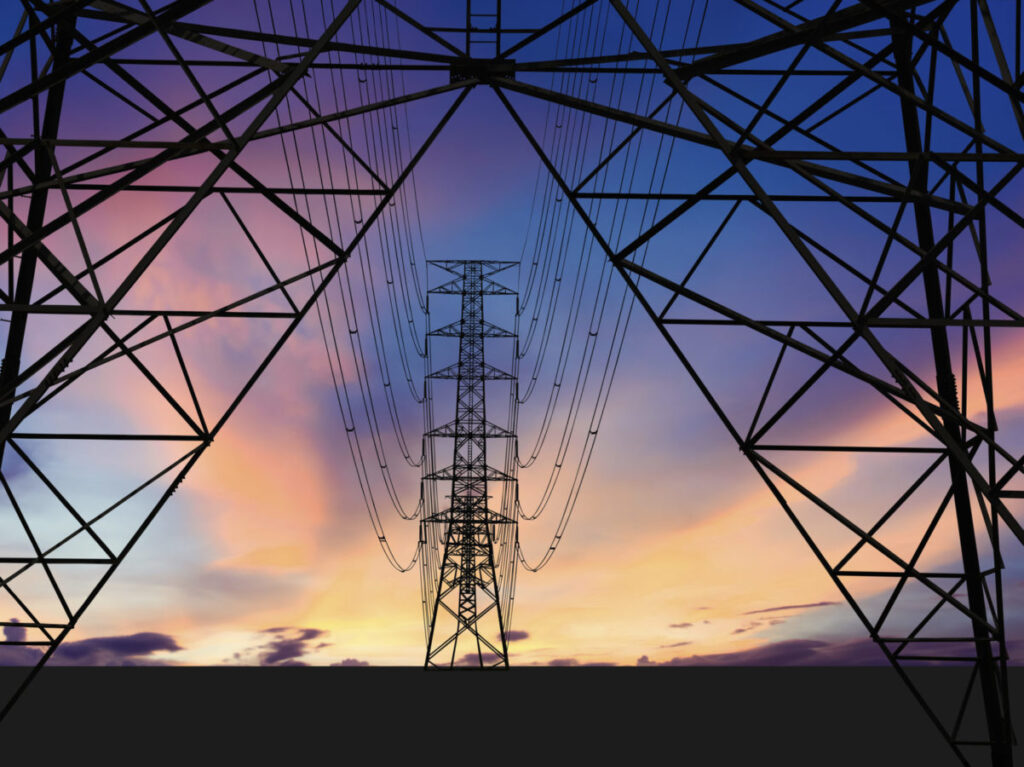
Could data hubs provide a life preserver for analytics teams thrown into the deep end with IoT data?
“Data hub” may be one of the more popular analytics buzzwords of 2019, quickly surpassing its relative and predecessor, the “data lake,” in mentions. But aside from the terminology, the actual function of the two concepts is proving to be the real differentiator.
As it turns out, while data lakes are good for storing raw data, it takes a lot of effort to make that data easily useful for applying advanced analytics like AI and machine learning. The data hub has been offered up as a data architecture that promises to quickly ingest, clean and label data, and then enable data scientists to use subsets of data to build, train, and deploy machine-learning models.
Most utilities today have either recently deployed, are in the process of implementing, or are at least considering a consolidated data solution for the purpose of analytics. For those in the early stages, should data hubs be on the list for consideration?
If they are hoping to build a foundation upon asset analytics and IoT, then the answer is yes.
Asset Hubs and IT/OT Convergence
For the last decade, utilities have been focused on IT/OT convergence, which itself is not easily defined. One way to look at the concept is how to effectively get operational personnel and systems to work with information technology personnel and systems for greater efficiency and reliability.
For asset management, utilities have largely struggled to marry near real-time data stored in operational systems, such as SCADA and DCS, with more static data stored in IT systems, such as work and asset management, GIS, enterprise resource planning and other applications.
According to one utility operational lead, “We have so much asset data in-house that we haven’t been able to utilize because it isn’t linked directly to assets. The more data you can draw on, the better the modeling results.”
Operational systems data plus sensor data (temperature, vibration, current, pressure, etc.) provide up-to-date data on asset behavior and conditions. The IT applications provide context – when the asset was last repaired, the criticality of the asset to operations, the asset hierarchy, and the cost of replacement. As more asset data becomes usable alongside the proliferation of IoT, utilities can drastically improve their ability to assess asset health, calculate risk, detect anomalies, and predict failures.
Utility Initiatives
It is not easy to find examples of utilities deploying data hubs. However, at least two utilities are “dipping a toe into the lake” to test out asset data hubs.
- Build your own asset hub. As part of a transmission asset management project, one large East Coast IOU is bringing together the foundational elements of data governance by building an intelligent (asset) data hub. The hub is designed with detailed business rules for each extract transform and load (ETL) and source system being integrated. It will clean/repair the data and support on-going data management. The plan is to bring together all relevant data and relate it to an in-service asset or documented failed asset for comprehensive analysis on a regular basis.
The project will apply best practices from rule-based engineering analytics and principles of physics with machine learning and artificial intelligence tools to provide actionable insights into what is likely to happen next and take the most effective mitigating action to change that course. Analytics are supplied by vendors.
- Access automated tools and services provided by vendors. Nova Scotia Power has recently chosen the Element AF Accelerator, a packaged solution combining Element’s AssetHub software with their engineering services and industrial data methodology to integrate, model and maintain operational and maintenance data that can be used across the enterprise for functions including asset maintenance and analytics. The asset hierarchy of the work and asset management system is applied to the data historian data in the data lake. Pre-built asset templates and data transformation tools are expected to reduce the time and effort to populate the asset hub.
First, NSP is tackling the task of providing users with contextual information sourced from asset performance management (APM) software, the data historian, maintenance management system, and engineering schematics. For example, a user viewing a draft fan in a certain functional location can view criticality, winding temperature, and inlet temperature as well as seeing displays of the motor and fan models, work orders and APM risks. Confidence in results produced by plant performance modeling software is expected to increase with additional data inputs made available through the asset data hub.
What to look for
It is not that utilities haven’t tried to bring asset data together in the past. However, there is an acknowledgment that the effectiveness of analytics and analytics-heavy asset performance management applications have been dragged down. It takes considerable effort to prepare quality data for ingestion.
Based on the experience of utilities that have taken the plunge into data hubs, others considering using this approach will want to look for solutions that will be able to document:
- Persistence of data resourced from operational systems. For example, when conditions of a piece of equipment change, the graph model of that piece of equipment changes.
- Utilization of data governance (retention, permissions, security, quality) in line with the industry standards
- Data transformation tools and pre-built equipment models that reduce the time it takes to build asset frameworks