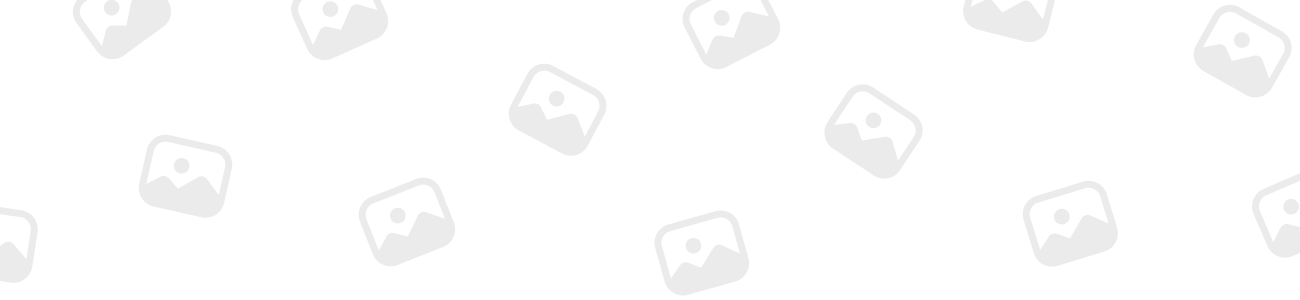
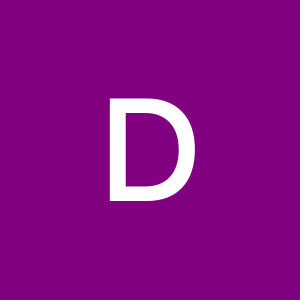
Data Science Community
In its simplest form, data science involves developing methods of recording, storing, and analyzing... View more
Unanswered Questions from August 16 Session – “Using Machine Learning to Detect Broken AMI Meters”
-
Unanswered Questions from August 16 Session – “Using Machine Learning to Detect Broken AMI Meters”
Hello Data Science Community Members,
We had an excellent session today, 8/16/22, during the Data Science Community Conversation by @Sarah and @Brad from The Energy Authority on “Using Machine Learning to Detect Broken AMI Meters”. Thanks so much to Sarah and Brad!
We had some great questions from members throughout the presentation, so many that we ran out of time to answer several questions. I sent these questions over to Sarah and Brad and they were gracious enough to provide answers. Please find the Q&A we missed included below.
Q: How did you deliver the model results to the end users? Feed the results to power BI? Or feed it to operational app for end users? @Qing
A: The results are delivered daily through the PowerBI report and weekly through an automated email. We’ve had discussions with other utilities about creating an automated process for work orders, but this set up is working well for this utility’s end users.Q: When you were creating the training data, did you use all the meter data or only the ones visited by truck team? @Huseyin
A: We only used meters that were visited by the field crews. They provide information about the field activity for the meter and we process that to classify it as either broken or not broken.Q: Did you feed time features to the model? If yes, how did you input them? @Bhanu
A: The granularity of the AMI data is daily, so we included date features but not time features.Q: Did you collect data from the workers actually going on site for a prospective recall? Did you include it in the modeling or planning? @Fernando
A: Yes, the historical field activity dataset contains information about what the workers found at the sites, so we used that to create the training dataset. It included information about the work done and comments made by the crews, which we used to classify the meter as broken or not broken. The logic for that classification isn’t perfect though, so I imagine there is a small amount of error in our training dataset from inaccuracies in the classification.Q: How did you adjust for seasonality? @Francis
A: We didn’t make any adjustments or transformations to remove seasonality, we just included features to try to capture changes in customer usage behavior due to seasonality.Q: How big was the dataset when implementing the models/training? @Pablo
A: After cleaning up the historical field activity/AMI data, we probably had about 25k meters with complete data in our final dataset. We split that 70/30 for training and testing, and then used incoming field activity data for additional model validation.Q: High usage related with frequent 0 reads seems like a revenue protection issue. Did you find that to be true? @Sashi Sridhar
A: Not always, some water meters are low granularity so my understanding is that they report 0 until the customer crosses a certain threshold and then they report usage. And as Francis mentioned, not all meters are being used every day
Please let us know if you have any additional questionsThanks!
Leslie
——————————
Leslie Cook
Membership & Digital Engagement Manager
Utility Analytics Institute (UAI)
719-203-8650, lcook@utilityanalytics.com
——————————
Sorry, there were no replies found.
Log in to reply.