Increasing grid reliability through AI and the power of prediction
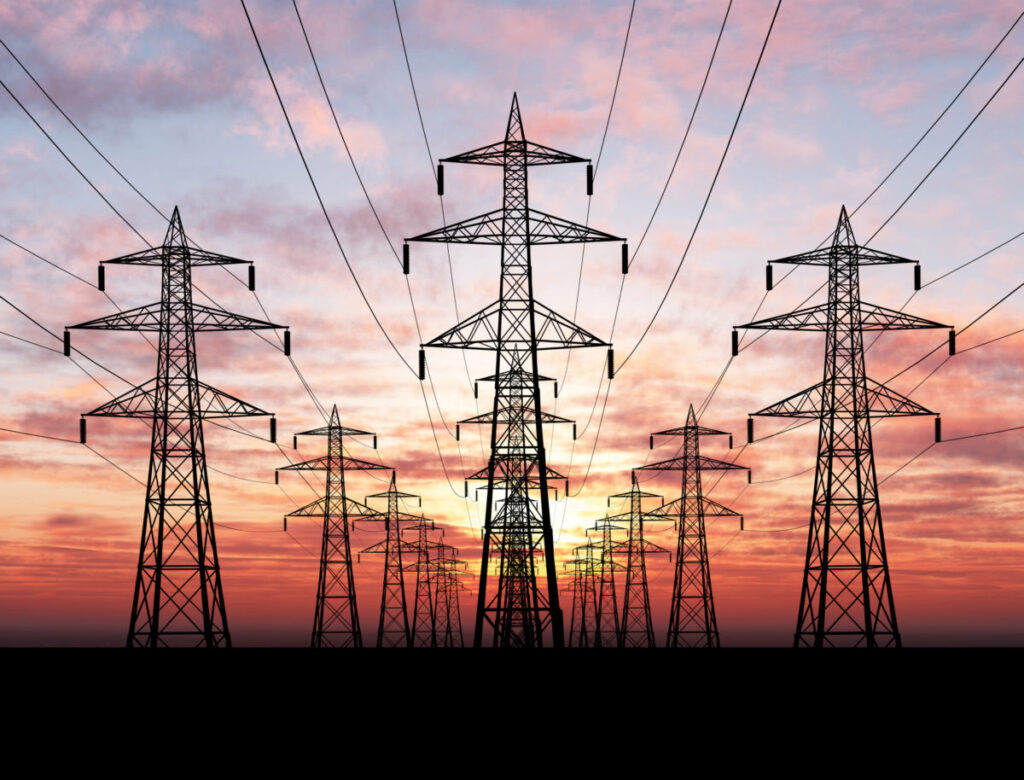
Tom Martin, formerly of PG&E and now Trove, discusses an AI data-driven path to outage prevention
Reliability – the ability to deliver uninterrupted power to residences and businesses across a service territory – is a top priority of every electric utility. It is the measure by which each is judged both by consumers and regulators, and achieving it economically is a pressing and universal challenge.
That’s because outages are hard to predict and expensive to prevent – they can occur anywhere, any time, and for any number of reasons across the grid. Common causes include equipment failure, weather-related events such as lightning strikes and downed limbs, and animal interference. To deal with this diffuse threat, electric utilities typically employ cadence-based maintenance measures, shoring up their assets on a prescribed timetable.
Yet this approach isn’t without its perils. First, because flattening load growth has O&M costs constantly in the cross-hairs, these outage-prevention measures are often the first to be postponed in response to short-term financial pressures, exposing utilities to greater financial and physical risks in the longer term. Second, while clearly the industry norm, their efficacy is in doubt. While “an ounce of prevention” may be worth “a pound of cure,” utilities are beginning to question whether doling out preventative measures broadly on a prescribed timetable is really the best way to achieve reliability.
One utility wanted to know for sure
A large electric utility serving millions of customers had already demonstrated the immediate benefits of teaming with TROVE Predictive Data Science in applying a risk-based approach to transmission reliability improvements across vegetation management, lightning protection, and avian guards, to name a few. For example, by using a combination of historic outages, topology, bird migration studies, and other data sources to identify the highest-risk assets for future avian reliability issues, TROVE had helped the utility reduce avian protection expenses by 75% (a three-month payback on the analytics effort).
This resounding success had made the utility eager to see if it could scale a predictive outage-prevention program to improve the reliability of an entire distribution region, one that had chronically lagged others in its service network.
Taking Measure of the Problem
Prior to TROVE, the utility had used a KPI common across electric utilities – customer minute interruptions (CMI)– to determine which poor performing circuits and regions were ripe for reliability improvements. And, in this under-performing region, that number was too high.
Armed with this data, the utility invested millions in rapid-response crews to reduce the duration of outages and new technology to quickly re-route power in the event of an outage, reducing the number of customers affected. Both performed admirably, but something unexpected happened – the KPI didn’t come down.
The utility turned to TROVE for help.
Upon further examination, one problem lay in the KPI itself. While CMI is key for understanding how the system as a whole is performing, it wasn’t designed to be an operational decision-making tool. It cannot provide insight into the drivers of poor reliability. Even more granular KPIs such as CAIDI, SAIDI, or SAIFI, which provide more targeted insights into duration and frequency of outages, are just reporting metrics and weren’t designed to provide insights into predicting and preventing outages.
Understanding how to actually invest in and improve reliability would be key, but doing so would require understanding the root causes of these outages – and some out-of-the-box thinking.
“While it would have been tempting to start developing predictive models from regional data, we were intent on understanding outages more broadly across the utility to inform a more comprehensive and universal fix,” said Jonah Keim, Senior Data Scientist at TROVE. “We started by examining years of historic outage data across the utility’s entire service area, drilling down into every power line and asking a lot of questions. These ranged from what time an outage occurred and how many people it affected to where the outage originated, the age, condition, and material of the conductors involved, the lengths and types of power lines, and even the topography of the land.”
Pulling from the utility’s own disparate data sources and augmenting them with additional vegetation, weather, topographical, and avian data, TROVE fleshed out more than 400 feeder attributes to qualify each historical outage, training its predictive models on this enhanced data set to determine which attributes or combination of attributes were indicators of potential future outages.
As a result, the utility can now run an outage “risk score” for all its grid assets across each circuit in its entire service territory.
Key Findings
By exploring the root causes of historical outages across the utility’s network using an enhanced data set and powerful AI and machine learning tools, TROVE has helped the utility develop a more strategic and precise risk-based approach to asset management and reliability, creating a new and improved “common language” for assessing risk and deploying capital. In just a few months, this predictive data science initiative has led to major improvements at the utility, including:
- A better metric to assess reliability performance. As part of traditional CAIDI and SAIDI system-level metrics, customer minute interruptions can be a useful KPI for utilities – it just wasn’t the right lens to address fixing this particular feeder, where a more granular metric would be more useful. As a result of its work with TROVE, the utility now uses a new “performance by circuit mile” metric to measure reliability. For added context, the feeder in the utility’s underperforming territory also happened to be the longest in the network. Longer feeders inherently have more problems, so simply saying, “My worst performing feeder will benefit the most from additional reliability measures” is not correct. Such feeders may be poor performing because of things that can’t be changed, such as feeder length. TROVE delivered a new way of looking at the problem to focus on identifying which “actionable” reliability measures would net the best results. Measures that are best for reporting aren’t necessarily best for taking action.
- A risk score for every asset. TROVE Solvers have provided the utility a way to easily score its grid assets according to risk of outage, helping the utility identify not only the hot spots in its network but also the specific drivers of that risk and the opportunity to direct capital investments accordingly based on risk instead of cadence. Applied to the underperforming region, the approach helped identify specific assets at higher risk of causing an outage, leading to the investment of capital there vs. into the prior cadence-based maintenance schedule. The end result delivers reduced outages, minimizes expensive and unplanned work, and shrinks one of the biggest customer “dissatisfiers.”
- A common, data-driven language for assessing risk and planning investment. Having successfully moved away from “system level” metrics for “feeder level” understanding, the utility is now embracing a common, data-driven language for assessing risk across its network. This data-driven way is shaping strategic planning – i.e. capital investment decisions – as well as informing tactical execution of the plan.
Tom Martin is TROVE’s Managing Director of Product, Energy & Utilities helping utilities become more data-driven and cost-effective in their decision making. Prior to TROVE, Tom led the Emerging Grid Technology at Pacific Gas & Electric leading pilot projects for new technology and analytics in support for PG&E’s Electric Operations as PG&E looked to reduce operational costs, improve safety, and increase reliability in support of grid modernization.