Utility Analytics 301
Utility Analytics 301: Machine Learning and Big Data Analytics for Power Systems & Smart Grid
The course provides background information, real-world development experience, and in-depth discussions of big data analytics and machine learning in power systems and smart grid. The value, velocity, volume, and variety of big data in smart grid will be discussed. The basics of machine learning algorithms such as unsupervised learning, supervised learning, reinforcement learning algorithms, graphical learning, and generative models will be covered and taught. Important real-world applications of big data analytics and machine learning in transmission system, distribution system, and electricity market will be presented and discussed.
-
Day 1 (4 hours)
- Self-Introduction
- Course Objectives
- Big Data in Power Systems & Smart Grid
- Overview of Machine Learning Applications in Smart Grid
- Overview of Machine Learning Algorithms
- Group Exercise: Selecting the Right Use Cases for ML in Power Systems and Smart Grid, Identify the Necessary Datasets for the Use Cases, Determine the Appropriate ML Algorithm
-
Day 2 (4 hours)
- Applications of Machine Learning in Distribution System
- Applications of Machine Learning in Electricity Market
- Group Discussion: Practical Implementation Challenges
-
Day 3 (4 hours)
- Applications of Machine Learning for End-Use Customers
- Applications of Machine Learning in Transmission System
- Applications of Machine Learning in Distribution System
- Group Discussion: Generative AI and its Applications in Electric Utility Business
Course Calendar
Utility Analytics 301: Machine Learning and Big Data Analytics for Power Systems & Smart Grid
Course Objectives
- Understand how to assess the business value of machine learning and big data analytics in smart grid
- Identify important machine learning and big data applications in smart grid
- Explain and selection of machine learning algorithms
- Learn about how to develop big data applications in smart grid
- Understand how to apply machine learning algorithms to solve problems in transmission system, distribution system, and electricity market
Appropriate Audience
This course is appropriate for the following audiences:
- Electric, combination utility, software provider, consulting company, proprietary trading firms, independent system operator.
- Engineers, data analysts, data scientists, business analysts, and managers.
- Distribution Planning, Distribution Engineering, Distribution Operators, Transmission Planning, Transmission Engineering, Transmission Operations, Customer Service.
- Utility professionals who are interested in machine learning and big data analytics in power systems and smart grid
- Utility Analytics 101 and 201 completers who want to continue advancing their in-depth knowledge of machine learning in power systems and smart grid
Prerequisites
- College- or university-level statistics and algebra or equivalent experience.
- Prior knowledge of machine learning and big data analytics is beneficial.
- It is recommended, but not required, to complete the UAI Utility Analytics 101 and/or 201 course(s).
Pricing
Virtual Training
In-Person Training
Virtual Training
UAI Member
-
Regular Pricing: $895
Non-Member
-
Regular Pricing: $1095
In-Person Training
UAI Member
-
Regular Pricing: $1895
Non-Member
-
Regular Pricing: $2395
Not a UAI member and interested in learning more? Contact our Membership Team!
UAI Utility Membership is at the organizational level and is designed to aid utilities looking to realize desired business outcomes using analytics. Membership benefits are centered around an experience that allows utility members to share insights, knowledge and practical application techniques.
UAI Utility Membership allows everyone with a stake in analytics to take the lead, get involved and start their journey to become a smarter utility analytics professional.
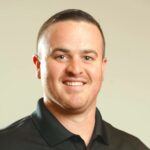
James Wingate
Membership Development Manager
jwingate@endeavorb2b.com
404-226-3756
Meet The Instructor
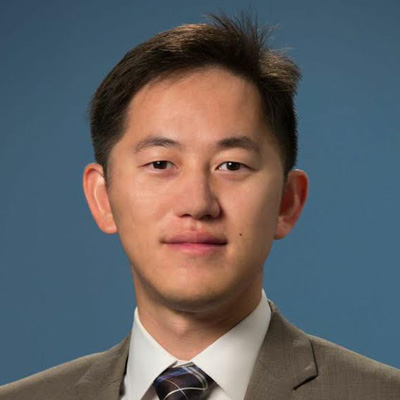
Dr. Nanpeng Yu
Professor and Vice Chair for Graduate Affairs, Department of Electrical and Computer Engineering
University of California, Riverside
From Our Students
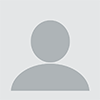
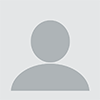